Recently, the 2023 CCF-Lenovo Blue Ocean Research Fund released the results of the evaluation of the fund topics, and the topic of "Information Understanding and Contextual Task Perception Modeling Methods Based on Multimodal Interaction" declared by Mingming Fan, Assistant Professor of the Computational Media and Arts Domain and the Internet of Things Thrust of the Hong Kong University of Science and Technology (Guangzhou), was awarded the funding.
The CCF-Lenovo Blue Ocean Research Fund is a research fund jointly established by CCF and Lenovo for scholars in Chinese universities, aiming to closely integrate the challenges of forward-looking technologies in industry with scientific research in universities, and to accelerate the realization of cutting-edge technologies in industry.
In 2023, CCF-Lenovo Blue Ocean Research Fund is directly facing the latest academic and industrial development, focusing on "Artificial Intelligence + Computing", focusing on forward-looking technologies and their application in real business scenarios, and releasing a number of cutting-edge technological challenges, with a funding rate of only 20%. Lenovo's technical team and CCF experts conducted a rigorous and comprehensive evaluation from the dimensions of challenge matching, topic innovation, technology leadership, and industrialization feasibility, and finally confirmed the funding of 10 topics. The following is the introduction of Prof. Fan Mingming's topic:
基于多模态交互的信息理解与情境任务感知建模方法
A Modeling Approach to Information Understanding and Contextual Task Perception Based on Multimodal Interaction
(English translation for reference only)
With the advancement of AI and IoT technologies, intelligent systems are equipped with powerful context-awareness capabilities, e.g., smartphones can provide automated services by sensing users' physical and social environments. However, facing the diversity of information and the complexity of task requirements, information processing faces great challenges.
The main technical difficulties include:
Multimodal Input Understanding, how to effectively process and extract knowledge from multimodal inputs such as text, images, and audio. Although deep learning models have been applied in these areas, it is time-consuming and labor-intensive to acquire a large amount of labeled data, and traditional models often ignore the correlation between different modalities, which affects the information fusion effect.
Context Awareness and Task Recognition, how to recognize users' task requirements based on the context they are in. Existing techniques mainly rely on rule matching and machine learning algorithms, but with a high demand for data annotation and insufficient characterization of dynamic changes and correlations between contexts and tasks.
The above technical challenges make it difficult for traditional assistant applications to effectively address user pain points.
With the development of AI technology, large models such as GPT-4 have shown strong capabilities in understanding and generating textual information.
Therefore, it is of great research significance and practical value to combine large models and human-computer interaction technologies in order to solve the problem of context-awareness and modeling based on multimodal data, and to enhance the capability of personal information assistants.
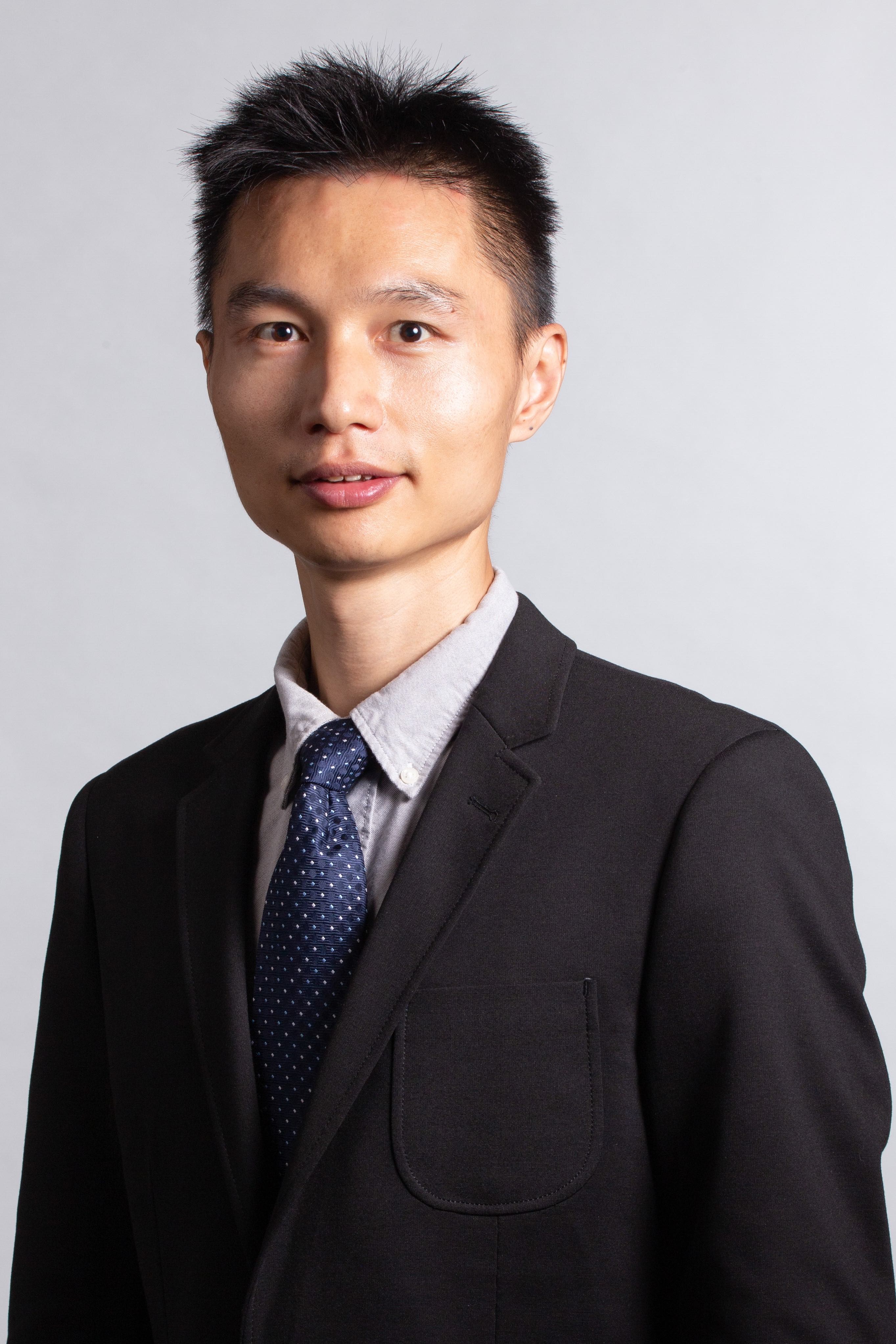